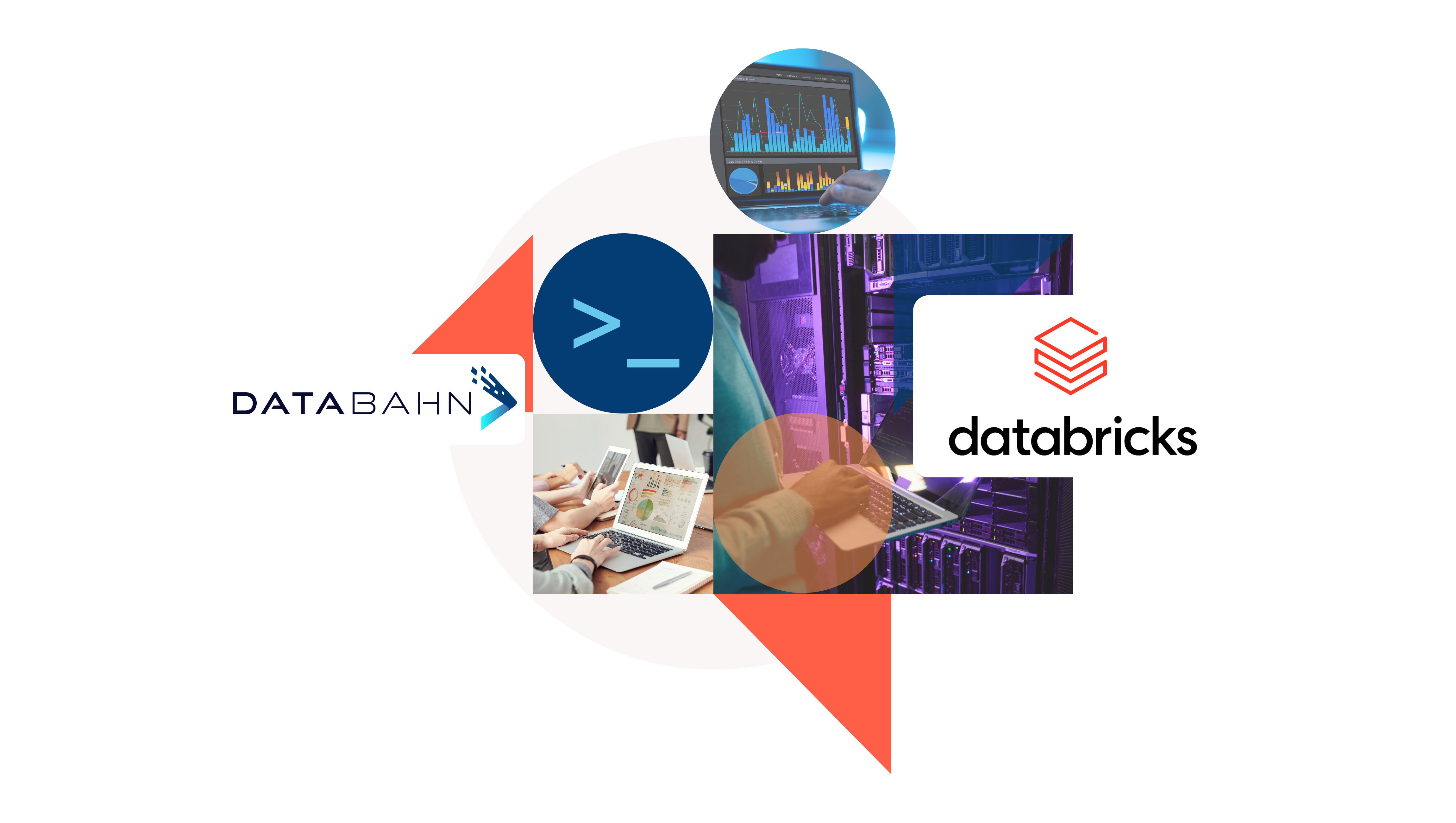
Data Orchestration and storage in Databricks’ Data Lakehouse
Use DataBahn’s Data Fabric to simplify data collection
Databricks is being chosen by many enterprises to be their data lake. Databricks’ Data Lakehouse allows for complex data science use cases, analytics, and ML operations with its Managed MLflow offering. Databricks’ ability to scale and manage large volumes of data and its extensive libraries with multi-language support makes it favored by large production enterprises in complex industries. However, data collection and ingestion continues to be complex. Centralized log ingestion requires customized data pipelines to ensure query performance, which has made some organizations delay their migration from monolithic data stores to modern, lakehouse-powered platforms such as Databricks.
Databahn helps Databricks users by streamlining data collection and ingestion and removing the burden of building customized integrations and pipelines, deploying staging locations, or managing data orchestration. It makes it easier for enterprise teams to sequester and efficiently route relevant data for analytics and processing, as well as sending less-relevant data to external tables and iceberg tables.
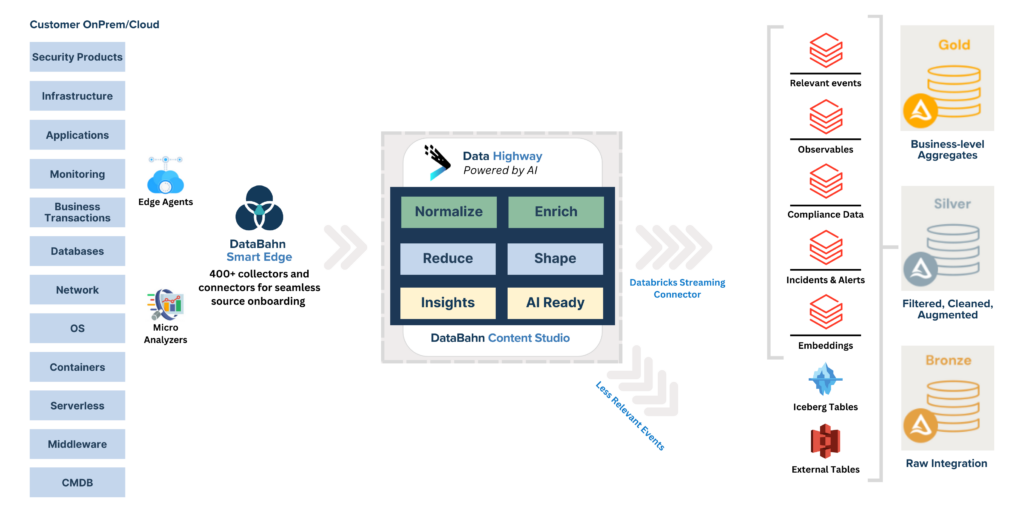