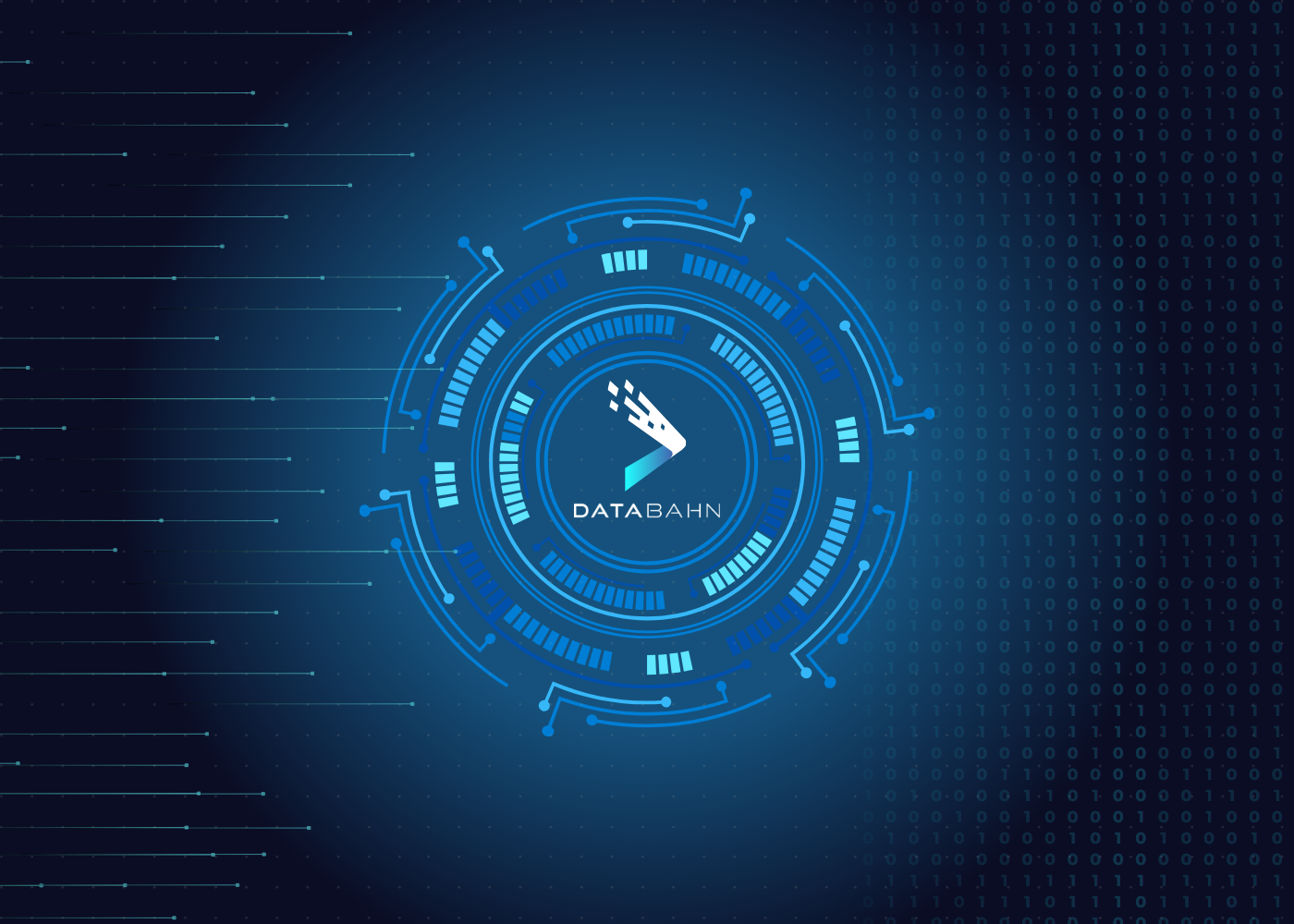
Telemetry Data Pipelines
and how they impact decision-making for enterprises
For effective data-driven decision-making, decision-makers must access accurate and relevant data at the right time. Security, sales, manufacturing, resource, inventory, supply chain, and other business-critical data help inform critical decisions. Today’s enterprises need to aggregate relevant data from around the world and various systems into a single location for analysis and presentation to leaders in a digestible format in real time for them to make these decisions effectively.
Why telemetry data pipelines matter
Today, businesses of all sizes need to collect information from various sources to ensure smooth operations. For instance, a modern retail brand must gather sales data from multiple storefronts across different locations, its website, and third-party sellers like e-commerce and social media platforms to understand how their products performed. It also helps inform decisions such as inventory, stocking, pricing, and marketing.
For large multi-national commercial enterprises, this data and its importance get magnified. Executives have to make critical decisions with millions of dollars at stake and in an accelerated timeline. They also have more complex and sophisticated systems with different applications and digital infrastructures that generate large amounts of data. Both old and new-age companies must build elaborate systems to connect, collect, aggregate, make sense of, and derive insights from this data.
What is a telemetry data pipeline?
Telemetry data encompasses various types of information captured and collected from remote and hard-to-reach sources. The term ‘telemetry’ originates from the French word ‘télémètre’, which means a device for measuring (“mètre”) data from afar (“télé”). In the context of modern enterprise businesses, telemetry data includes application logs, events, metrics, and performance indicators which provide essential information that helps run, maintain, optimize, and systems and operations.
A telemetry pipeline, as the name implies, is the infrastructure that collects and moves the data from the source to the destination. But a telemetry data pipeline doesn’t just move data; it also aggregates and processes this data to make it usable, and routes it to the necessary analytics or security destinations where it can be used by leaders to make important decisions.
Core functions of a telemetry data pipeline
Telemetry data pipelines have 3 core functions:
- Collecting data from multiple sources;
- Processing and preparing the data for analysis; and
- Transferring the data to the appropriate storage destination.
DATA COLLECTION
The first phase of a data pipeline is collecting data from various sources. These sources can include products, applications, servers, datasets, devices, and sensors, and they can be spread across different networks and locations. The collection of this data from these different sources and moving them towards a central repository is the first part of the data lifecycle.
Challenges: With the growing number of sources, IT and data teams find it difficult to integrate new ones. API-based integrations can take between four to eight weeks for an enterprise data engineering team, placing significant demands on technical engineering bandwidth. Monitoring and tracking sources for anomalous behavior, identifying blocked data pipelines, and ensuring the seamless flow of telemetry data are major pain points for enterprises. With data volumes growing at ~30% Y-o-Y, being able to scale data collection to manage spikes in data flow is an important problem for engineering teams to solve, but they don’t always have the time and effort to invest in such a project.
DATA PROCESSING & PREPARATION
The second phase of a data pipeline is aggregating the data, which requires multiple data operations such as cleansing, de-duplication, parsing, and normalization. Raw data is not suitable for leaders to make decisions, and it needs to be aggregated from different sources. Data from different sources have to be turned into the same format, stitched together for correlation and enrichment, and prepared to be further refined for further insights and decision-making.
Challenges: Managing the different formats and parsing it can get complicated; and with many enterprises building or having built custom applications, parsing and normalizing that data is challenging. Changing log and data schemas can create cascading failures in your data pipeline. Then there are challenges such as identifying and masking sensitive data and quarantining it to protect PII from being leaked.
DATA ROUTING
The final stage is taking the data to its intended destination – a data lake or lakehouse, a cloud storage service, or an observability or security tool. For this, data has to be put into a specific format and has to be optimally segregated to avoid the high cost of the real-time analysis tools.
Challenges: Different types of telemetry data have different values, and segregating the data optimally to manage and reduce the cost of expensive SIEM and observability tools is high priority for most enterprise data teams. The ‘noise’ in the data also causes an increase in alerts and makes it harder for teams to find relevant data in the stream coming their way. Unfortunately, segregating and filtering the data optimally is difficult as engineers can’t predict what data is useful and what data isn’t. Additionally, the increasing volume of data with the stagnant IT budget means that many teams are making sub-optimal choices of routing all data from some noisy sources into long-term storage, meaning that some insights are lost.
How can we make telemetry data pipelines better?
Organizations today generate terabytes of data daily and use telemetry data pipelines to move the data in real-time to derive actionable insights that inform important business decisions. However, there are major challenges in building and managing telemetry data pipelines, even if they are indispensable.
Agentic AI solves for all these challenges and is capable of delivering greater efficiency in managing and optimizing telemetry data pipeline health. An agentic AI can –
- Discover, deploy, and integrate with new data sources instantly;
- Parse and normalize raw data from structured and unstructured sources;
- Track and monitor pipeline health; be modular and sustain loss-less data flow;
- Identify and quarantine sensitive and PII data instantly;
- Manage and fix for schema drift and data quality;
- Segregate and evaluate data for storage in long-term storage, data lakes, or SIEM/observability tools
- Automate the transformation of data into different formats for different destinations;
- Save engineering team bandwidth which can be deployed on more strategic priorities
Curious about how agentic AI can solve your data problems? Get in touch with us to explore Cruz, our agentic AI data-engineer-in-a-box to solve your telemetry data challenges.
Related Posts
Reduced Alert Fatigue: 50% Log Volume Reduction with AI-powered log prioritization
Discover how AI can reduce alert fatigue with intelligent log prioritization in Microsoft Sentinel
Identity Data Management – how DataBahn solves the ‘first-mile’ data challenge
Find out why telemetry data is important and how you can collect it better using…
Telemetry Data Pipelines – and how they impact decision-making for enterprises
Find out why telemetry data is important and how you can collect it better using…
Sentinel best practices: how SOCs can optimize Sentinel costs & performance
Microsoft Sentinel best practices How SOCs can optimize Sentinel costs & performance Enterprises and security…