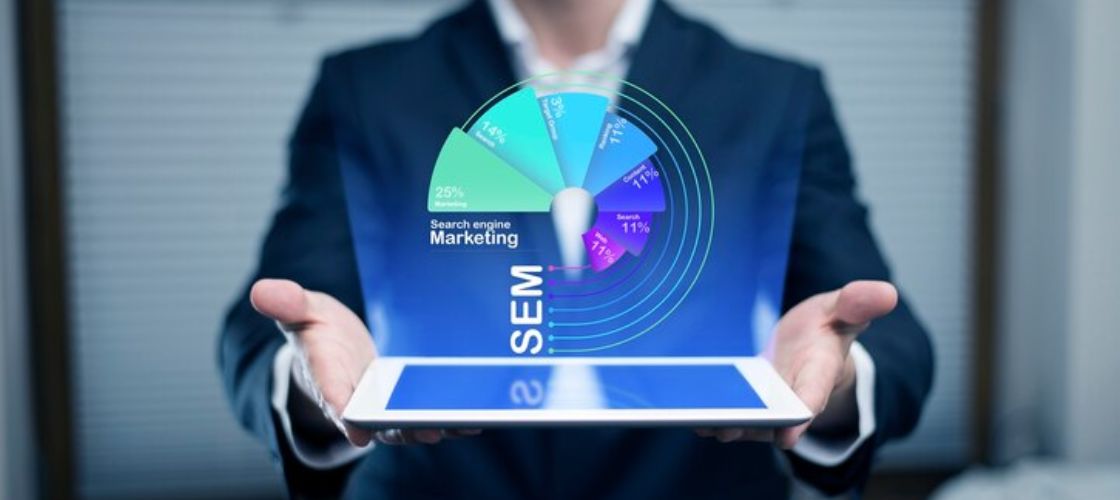
Maximizing Data Collection Efficiency At The Edge
As organizations embrace edge computing for real-time data processing, the demand for effective log filtering at the edge has surged. However, deploying agents on diverse assets introduces complexities related to resource utilization, rule management, and the need for scalable solutions. Agents, while effective, pose resource constraints and operational hurdles.
Challenges
Resource Strain
Agents demand memory and CPU, directly impacting asset (host) resources. For instance, as filtering rules grow complex, more compute resources are required, potentially leading to performance degradation or in extreme cases, dropped events. Example: A security agent exhausts resources on a critical server, dropping events during a high-risk security incident.
Management Complexity
A one-size-fits-all solution is unattainable. Managing rules across diverse assets, asset groups, Group Policy Objects (GPO), and Configuration Management Database (CMDB) settings becomes intricate. Example: Configuring unique filtering rules for different asset groups within a large enterprise is cumbersome, leading to misconfigurations and security risks.
Forensic Dilemma
Dropping events for future forensic needs creates complexities. For instance, during an investigation, dropped events due to resource limits hinder the security team’s ability to reconstruct a comprehensive timeline of incidents.
Operational
Dependency Teams are at odds over agent deployment, introducing dependencies and slowing down change implementation. Example: The security team requires a rule change for enhanced threat detection, but the change needs approval from multiple teams, delaying critical security updates and leaving the organization vulnerable.
Scaling Challenges
Solutions without agents struggle to scale the volume of data generated at the edge. Example: An agent-less solution attempting to fetch data on demand fails to keep up with real-time events during a sudden surge in network activity, causing data gaps.
Proposed Solutions
Solution-Agnostic Data Collection
Introduce a log collection layer that is agnostic to specific solutions, fetching all available data from hosts to a centralized location. This allows minimal impact at the edge while ensuring all the events are collected.
Mesh Style Distributed Log Collection Architecture
A mesh or a distributed data collection architecture eliminates ongoing dependencies such as manual interference to scale up or balance log collection workloads. This ensures resources allocated for collection are optimally used and ensures data resiliency at the collection layer.
Centralized Orchestration Engine
Introduce a centralized orchestration engine for data ingestion, forking and delivery. This engine collects data from hosts, appliances, applies filtering, classification, and aggregation processes all while optimizing the resources assigned to it.
Introducing DataBahn Smart Edge
A Highly Scalable and Resilient Data Collection at the Edge
DataBahn’s Smart Edge Service Mesh is designed to offer
- Extensive Connectivity: Offers a built-in library of connectors for seamless data integration.
- Cost Efficiency: Edge Analyzers significantly reduce cloud-cloud data egress costs.
- Unparalleled Data Collection Performance: Delivers performance that is 10x higher than traditional SIEM collectors.
- Simplified Integration: Features Auto Discovery for easier data integration and collection.
- Resilient Architecture: Utilizes a mesh design to ensure high resiliency and scalability.
- Flexible Deployment: Smart Edge Fleets can be deployed in both On-prem and Cloud environments, integrating natively with the existing agent ecosystem.
- Versatile Data Handling: Destination-agnostic to meet diverse data collection needs for SIEMs, Data Lakes, and Cold Storage.
Benefits include –
- Prevents data loss associated with traditional SIEM log forwarders and syslog relay servers.
- Enables decoupling of data collection from SIEM, avoiding vendor lock-in and fostering flexibility.
- Reduces data transfer costs between cloud environments.
- Eliminates the need for custom code or scripts for data collection, streamlining operations.
- Ensures high scalability and resilience to support growing data demands.
In conclusion, the challenges of edge log filtering demand a strategic shift. The proposed centralized data collection engine offers an efficient and scalable solution, addressing resource constraints, management complexities, and operational dependencies. By embracing this approach, organizations can streamline log filtering, enhance security, and adapt to the dynamic landscape of edge computing. By ensuring each layer is solution agnostic, it allows end users to bring best of breed solutions to each stage of the data collection and processing layer for security & observability.