The DataBahn blog
The latest articles, news, blogs and learnings from Databahn

.png)
In September 2022, cybercriminals accessed, encrypted, and stole a substantial amount of data from Suffolk County’s IT systems, which included personally identifiable information (PII) of county residents, employees, and retirees. Although Suffolk County did not pay the ransom demand of $2.5 million, it ultimately spent $25 million to address and remediate the impact of the attack.
Members of the county’s IT team reported receiving hundreds of alerts every day in the weeks leading up to the attack. Several months earlier, frustrated by the excessive number of unnecessary alerts, the team redirected the notifications from their tools to a Slack channel. Although the frequency and severity of the alerts increased leading up to the September breach, the constant stream of alerts wore the small team down, leaving them too exhausted to respond and distinguish false positives from relevant alerts. This situation created an opportunity for malicious actors to successfully circumvent security systems.
The alert fatigue problem
Today, cybersecurity teams are continually bombarded by alerts from security tools throughout the data lifecycle. Firewalls, XDRs/EDRs, and SIEMs are among the common tools that trigger these alerts. In 2020, Forrester reported that SOC teams received 11,000 alerts daily, and 55% of cloud security professionals admitted to missing critical alerts. Organizations cannot afford to ignore a single alert, yet alert fatigue (and an overwhelming number of unnecessary alerts) causes SOCs to miss up to 30% of security alerts that go uninvestigated or are completely overlooked.
While this creates a clear cybersecurity and business continuity problem, it also presents a pressing human issue. Alert fatigue leads to cognitive overload, emotional exhaustion, and disengagement, resulting in stress, mental health concerns, and attrition. More than half of cybersecurity professionals cite their workload as the primary source of stress, two-thirds reported experiencing burnout, and over 60% of cybersecurity professionals surveyed stated it contributed to staff turnover and talent loss.
Alert fatigue poses operational challenges, represents a critical security risk, and truly becomes an adversary of the most vital resource that enterprises rely on for their security — SOC professionals doing their utmost to combat cybercriminals. SOCs are spending so much time and effort triaging alerts and filtering false positives that there’s little room for creative threat hunting.
Data is the problem – and the solution
Alert fatigue is a result, not a root cause. When these security tools were initially developed, cybersecurity teams managed gigabytes of data each month from a limited number of computers on physically connected sites. Today, Security Operations Centers (SOCs) are tasked with handling security data from thousands of sources and devices worldwide, which arrive through numerous distinct devices in various formats. The developers of these devices did not intend to simplify the lives of security teams, and the tools they designed to identify patterns often resemble a fire alarm in a volcano. The more data that is sent as an input to these machines, the more likely they are to malfunction – further exhausting and overwhelming already stretched security teams.
Well-intentioned leaders advocate for improved triaging, the use of automation, refined rules to reduce false-positive rates, and the application of popular technologies like AI and ML. Until we can stop security tools from being overwhelmed by large volumes of unstructured, unrefined, and chaotic data from diverse sources and formats, these fixes will be band aids on a gaping wound.
The best way to address alert fatigue is to filter out the data being ingested into downstream security tools. Consolidate, correlate, parse, and normalize data before it enters your SIEM or UEBA. If it isn’t necessary, store it in blob storage. If it’s duplicated or irrelevant, discard it. Don’t clutter your SIEM with poor data so it doesn’t overwhelm your SOC with alerts no one requested.
How Databahn helps
At DataBahn, we help enterprises cut through cybersecurity noise with our security data pipeline solution, which works around the clock to:
1. Aggregates and normalizes data across tools and environments automatically
2. Uses AI-driven correlation and prioritization
3. Denoises the data going into the SIEM, ensuring more actionable alerts with full context
SOCs using DataBahn aren’t overwhelmed with alerts; they only see what’s relevant, allowing them to respond more quickly and effectively to threats. They are empowered to take a more strategic approach in managing operations, as their time isn’t wasted triaging and filtering out unnecessary alerts.
Organizations looking to safeguard their systems – and protect their SOC members – should shift from raw alert processing to smarter alert management, driven by an intelligent pipeline which combines automation, correlation, and transformation that filters out the noise and combats alert fatigue.
Interested in saving your SOC from alert fatigue? Contact DataBahn
In the past, we've written about how we solve this problem for Sentinel. You can read more here: AI-powered Sentinel Log Optimization
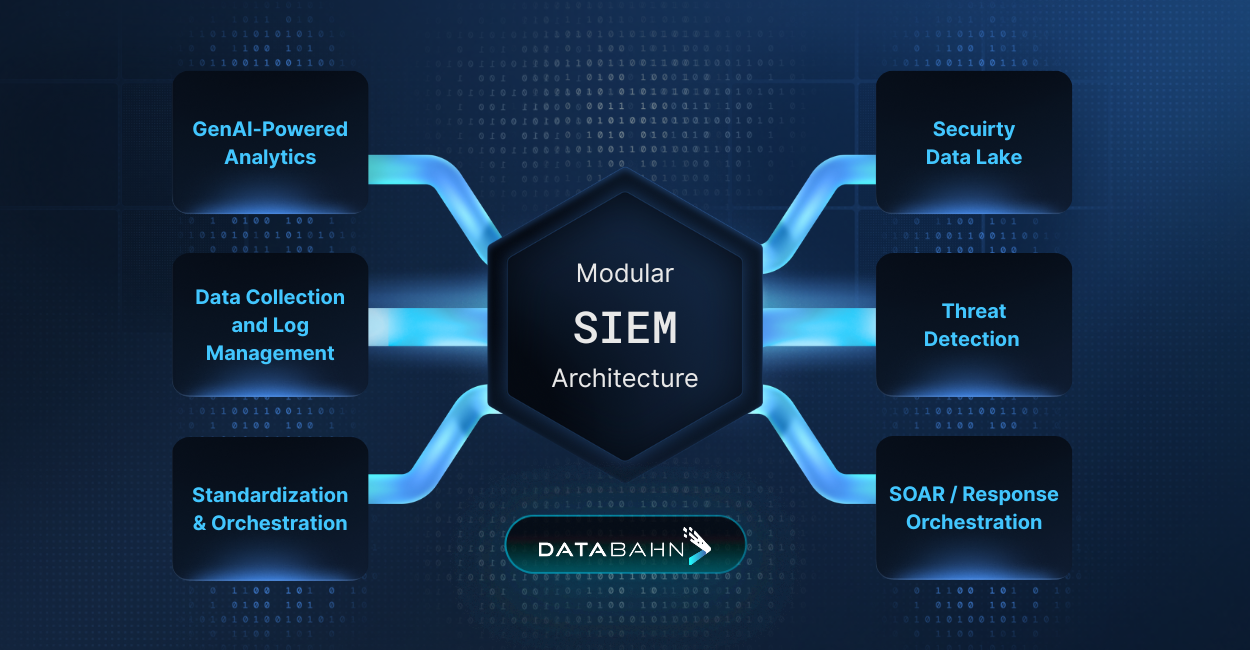
I see a lot more organizations head towards Headless Cyber Architecture. Traditionally, cybersecurity teams relied on one massive tool: the SIEM. For years, Cyber security orgs funneled all their cyber data into it; not because it was optimal, but because it was the compliance checkbox.
That’s how SIEMs earned their core seat at the table. Over time, SIEMs evolved from a log aggregator into something more sophisticated: UEBA ->Security Analytics -> and now, increasingly, SaaS-based platforms to more AI SOC. But there’s a catch—in this model, you don’t truly own your data. It lives in the vendor’s ecosystem, locked into their proprietary format rather than an open standard.
You end up paying for storage, analytics, and access to your own telemetry—creating a cycle of dependency and vendor lock-in.
But the game is changing. What’s New?
SIEMs are not going away; they remain mission-critical. But they’re no longer the sole destination for all cyber data. Instead, they are being refocused: They now consume only Security-Relevant Data (SRDs)—purposefully curated feeds for advanced threat detection, correlation, and threat chaining. Nearly 80% of organizations have only integrated baseline telemetry—firewalls, endpoints, XDRs, and the like. But where’s the visibility into mission-critical apps? Your plant data? Manufacturing systems? The rest of your telemetry often remains siloed, unparsed, and not in open, interoperable formats like OTEL or OCSF.
The shift is this : It’s now flowing into your Security Data Lake (SDL)—parsed, normalized, enriched with context like threat intel, HR systems, identity, and geo signals. This data increasingly lives in your environment: Databricks. Snowflake. Amazon Web Services (AWS), Microsoft Azure, Google Cloud, Hydrolix.
With this shift, a new category is exploding: headless cybersecurity products—tools that sit on top of your data rather than ingesting it.
· Headless SIEMs: Built for detection, not data hoarding.
· Headless Vulnerability Analytics: Operating directly on vuln data inside your SDL.
· Headless Data Science: ML models run atop your lake, no extraction needed.
· Soon: Headless IAM & Access Analytics: Compliance and reporting directly from where access logs reside.
These solutions don’t route your data out—they bring their algorithm to your lake. This flips the control model.
To Get There: The Data Pipeline Must Evolve
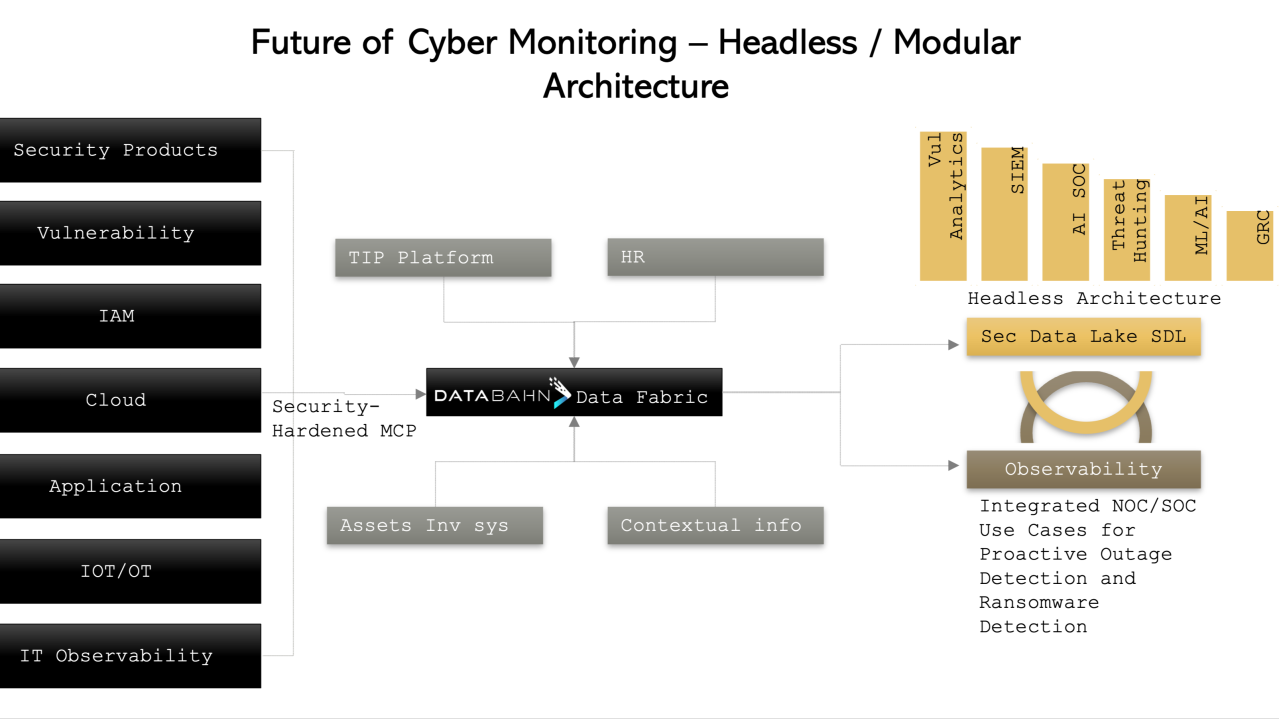
What’s needed is an independent platform purpose-built for streaming ETL and pipeline management, the connective tissue that moves, filters, and enriches your telemetry in real time. A platform that’s-
· Lightweight and modular—drop a node anywhere to start collecting from a new business unit or acquisition.
· Broadly integrated—connecting with thousands of systems to maximize visibility.
· Smart at filtering—removing up to 60%-80% of Non-Security Data (NSDs) that bloats your SIEM
· Enrichment-first—applying threat intel, identity, geo, and other contextual data before forwarding to your Security Data Lake (SDL) and SIEM. Remember, analysts spend valuable time manually stitching together context during investigations. Pre-enriched data dramatically reduces that effort—cutting investigation time, improving accuracy, and accelerating response.
· AI-ready—feeding clean, contextualized data into your models, reducing noise and improving MTTD/MTTR. Also helps desanitize sensitive information leaving your environment.
· Insightful in motion—offering real-time observability as data flows through the pipeline.
In short, the pipeline becomes the foundation for modern security architecture and the fuel for your AI-driven transformation.
With this shift, a new category is exploding: headless cybersecurity products—tools that sit on top of your data rather than ingesting it.
Bottom Line : We’re entering a new era where
· SIEMs do less ingestion, more detection
· Data lakes become the source of truth, enriched, stored in your format
· Vendors no longer take your data—they work on top of it
· Security teams get flexibility, visibility, and control—without the lock-in
This is the rise of modular, headless cybersecurity—
This is the rise of modular, headless cybersecurity, where your data stays yours. Their analytics run where you want and computing happens on your terms, all while you have complete control over your data.

DataBahn recognized as leading vendor in SACR 2025 Security Data Pipeline Platforms Market Guide
As security operations become more complex and SOCs face increasingly sophisticated threats, the data layer has emerged as the critical foundation. SOC effectiveness now depends on the quality, relevance, and timeliness of data it processes; without a robust data layer, SIEM-based analytics, detection, and response automation crumble under the deluge of irrelevant data and unreliable insights.
Recognizing the need to engage with current SIEM problems, security leaders are adopting a new breed of security data tools known as Security Data Pipeline Platforms. These platforms sit beneath the SIEM, acting as a control plane for ingesting, enriching, and routing security data in real time. In its 2025 Market Guide, SACR explores this fast-emerging category and names DataBahn among the vendors leading this shift.

Understanding Security Data Pipelines: A New Approach
The SACR report highlights this breaking point: organizations typically collect data from 40+ security tools, generating terabytes daily. This volume overwhelms legacy systems, creating three critical problems:
First, prohibitive costs force painful tradeoffs between security visibility and budget constraints. Second, analytics performance degrades as data volumes increase. Finally, security teams waste precious time managing infrastructure rather than investigating threats.
Fundamentally, security data pipeline platforms partially or fully resolve the data volume problems with differing outcomes and performance. DataBahn decouples collection from storage and analysis, automates and simplifies data collection, transformation, and routing. This architecture reduces costs while improving visibility and analytic capabilities—the exact opposite of the traditional, SIEM-based approach.
AI-Driven Intelligence: Beyond Basic Automation
The report examines how AI is reshaping security data pipelines. While many vendors claim AI capabilities, few have integrated intelligence throughout the entire data lifecycle.
DataBahn's approach embeds intelligence at every layer of the security data pipeline. Rather than simply automating existing processes, our AI continually optimizes the entire data journey—from collection to transformation to insight generation.
This intelligence layer represents a paradigm shift from reactive to proactive security, moving beyond "what happened?" to answering "what's happening now, and what should I do about it?"
Take threat detection as an example: traditional systems require analysts to create detection rules based on known patterns. DataBahn's AI continually learns from your environment, identifying anomalies and potential threats without predefined rules.
The DataBahn Platform: Engineered for Modern Security Demands
In an era where security data is both abundant and complex, DataBahn's platform stands out by offering intelligent, adaptable solutions that cater to the evolving needs of security teams.
Agentic AI for Security Data Engineering: Our agentic AI, Cruz, automates the heavy lifting across your data pipeline—from building connectors to orchestrating transformations. Its self-healing capabilities detect and resolve pipeline issues in real-time, minimizing downtime and maintaining operational efficiency.
Intelligent Data Routing and Cost Optimization: The platform evaluates telemetry data in real-time, directing only high-value data to cost-intensive destinations like SIEMs or data lakes. This targeted approach reduces storage and processing costs while preserving essential security insights.
Flexible SIEM Integration and Migration: DataBahn's decoupled architecture facilitates seamless integration with various SIEM solutions. This flexibility allows organizations to migrate between SIEM platforms without disrupting existing workflows or compromising data integrity.
Enterprise-Wide Coverage: Security, Observability, and IoT/OT: Beyond security data, DataBahn's platform supports observability, application, and IoT/OT telemetry, providing a unified solution for diverse data sources. With 400+ prebuilt connectors and a modular architecture, it meets the needs of global enterprises managing hybrid, cloud-native, and edge environments.

Next-Generation Security Analytics
One of DataBahn’s standout features highlighted by SACR is our newly launched "insights layer”—Reef. Reef transforms how security professionals interact with data through conversational AI. Instead of writing complex queries or building dashboards, analysts simply ask questions in natural language: "Show me failed login attempts for privileged users in the last 24 hours" or "Show me all suspicious logins in the last 7 days"
Critically, Reef decouples insight generation from traditional ingestion models, allowing Security analysts to interact directly with their data, gain context-rich insights without cumbersome queries or manual analysis. This significantly reduces the mean time to detection (MTTD) and response (MTTR), allowing teams to prioritize genuine threats quickly.
Moving Forward with Intelligent Security Data Management
DataBahn's inclusion in the SACR 2025 Market Guide affirms our position at the forefront of security data innovation. As threat environments grow more complex, the difference between security success and failure increasingly depends on how effectively organizations manage their data.
We invite you to download the complete SACR 2025 Market Guide to understand how security data pipeline platforms are reshaping the industry landscape. For a personalized discussion about transforming your security operations, schedule a demo with our team. Click here
In today's environment, your security data should work for you, not against you.
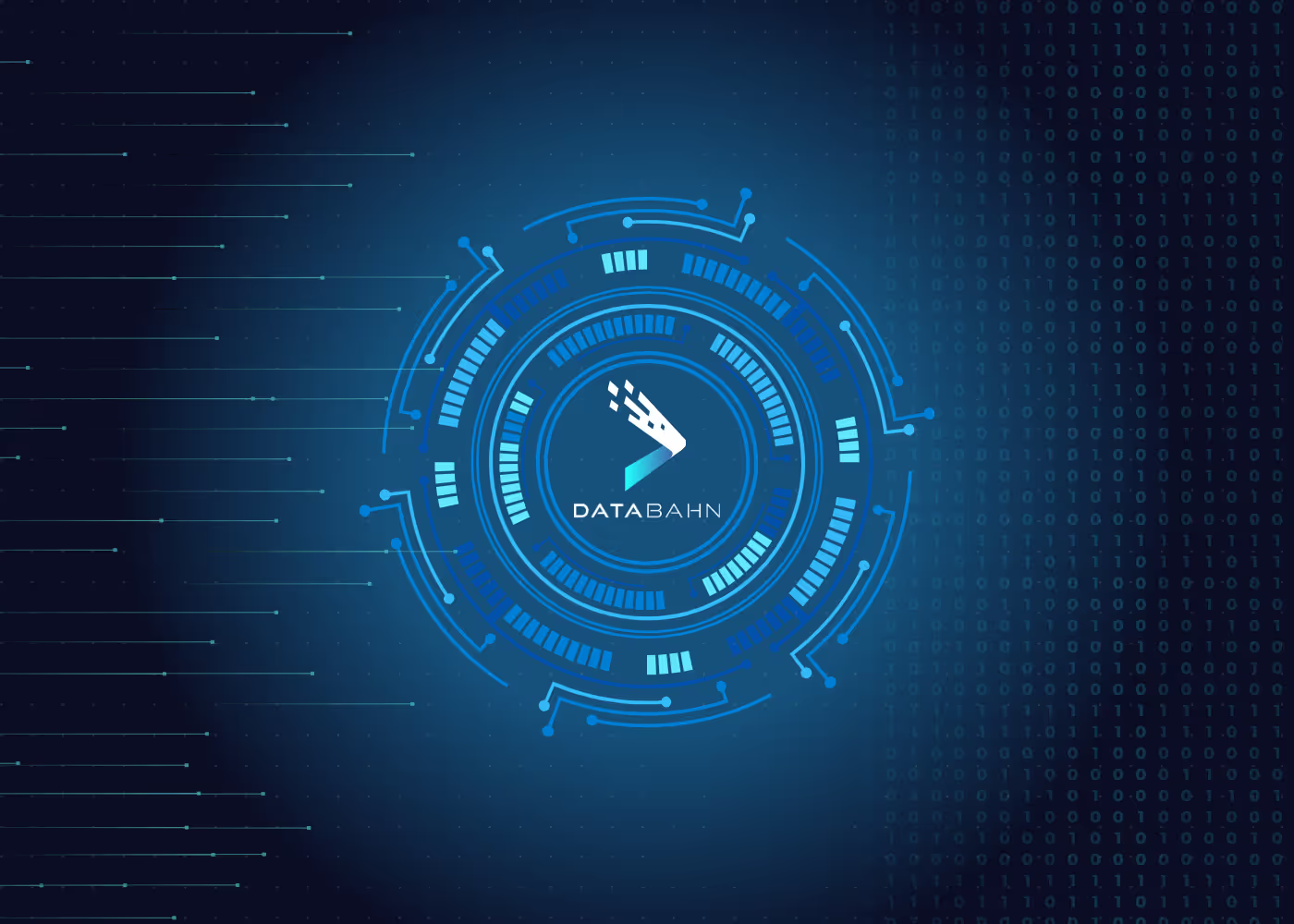
Telemetry Data Pipelines
and how they impact decision-making for enterprises
For effective data-driven decision-making, decision-makers must access accurate and relevant data at the right time. Security, sales, manufacturing, resource, inventory, supply chain, and other business-critical data help inform critical decisions. Today’s enterprises need to aggregate relevant data from around the world and various systems into a single location for analysis and presentation to leaders in a digestible format in real time for them to make these decisions effectively.
Why telemetry data pipelines matter
Today, businesses of all sizes need to collect information from various sources to ensure smooth operations. For instance, a modern retail brand must gather sales data from multiple storefronts across different locations, its website, and third-party sellers like e-commerce and social media platforms to understand how their products performed. It also helps inform decisions such as inventory, stocking, pricing, and marketing.
For large multi-national commercial enterprises, this data and its importance get magnified. Executives have to make critical decisions with millions of dollars at stake and in an accelerated timeline. They also have more complex and sophisticated systems with different applications and digital infrastructures that generate large amounts of data. Both old and new-age companies must build elaborate systems to connect, collect, aggregate, make sense of, and derive insights from this data.
What is a telemetry data pipeline?
Telemetry data encompasses various types of information captured and collected from remote and hard-to-reach sources. The term ‘telemetry’ originates from the French word ‘télémètre’, which means a device for measuring (“mètre”) data from afar (“télé”). In the context of modern enterprise businesses, telemetry data includes application logs, events, metrics, and performance indicators which provide essential information that helps run, maintain, and optimize systems and operations.
A telemetry pipeline, as the name implies, is the infrastructure that collects and moves the data from the source to the destination. But a telemetry data pipeline doesn’t just move data; it also aggregates and processes this data to make it usable, and routes it to the necessary analytics or security destinations where it can be used by leaders to make important decisions.
Core functions of a telemetry data pipeline
Telemetry data pipelines have 3 core functions:
- Collecting data from multiple sources;
- Processing and preparing the data for analysis; and
- Transferring the data to the appropriate storage destination.
DATA COLLECTION
The first phase of a data pipeline is collecting data from various sources. These sources can include products, applications, servers, datasets, devices, and sensors, and they can be spread across different networks and locations. The collection of this data from these different sources and moving them towards a central repository is the first part of the data lifecycle.
Challenges: With the growing number of sources, IT and data teams find it difficult to integrate new ones. API-based integrations can take between four to eight weeks for an enterprise data engineering team, placing significant demands on technical engineering bandwidth. Monitoring and tracking sources for anomalous behavior, identifying blocked data pipelines, and ensuring the seamless flow of telemetry data are major pain points for enterprises. With data volumes growing at ~30% Y-o-Y, being able to scale data collection to manage spikes in data flow is an important problem for engineering teams to solve, but they don’t always have the time and effort to invest in such a project.
DATA PROCESSING & PREPARATION
The second phase of a data pipeline is aggregating the data, which requires multiple data operations such as cleansing, de-duplication, parsing, and normalization. Raw data is not suitable for leaders to make decisions, and it needs to be aggregated from different sources. Data from different sources have to be turned into the same format, stitched together for correlation and enrichment, and prepared to be further refined for further insights and decision-making.
Challenges: Managing the different formats and parsing it can get complicated; and with many enterprises building or having built custom applications, parsing and normalizing that data is challenging. Changing log and data schemas can create cascading failures in your data pipeline. Then there are challenges such as identifying and masking sensitive data and quarantining it to protect PII from being leaked.
DATA ROUTING
The final stage is taking the data to its intended destination – a data lake or lakehouse, a cloud storage service, or an observability or security tool. For this, data has to be put into a specific format and has to be optimally segregated to avoid the high cost of the real-time analysis tools.
Challenges: Different types of telemetry data have different values, and segregating the data optimally to manage and reduce the cost of expensive SIEM and observability tools is high priority for most enterprise data teams. The ‘noise’ in the data also causes an increase in alerts and makes it harder for teams to find relevant data in the stream coming their way. Unfortunately, segregating and filtering the data optimally is difficult as engineers can't predict what data is useful and what data isn’t. Additionally, the increasing volume of data with the stagnant IT budget means that many teams are making sub-optimal choices of routing all data from some noisy sources into long-term storage, meaning that some insights are lost.
How can we make telemetry data pipelines better?
Organizations today generate terabytes of data daily and use telemetry data pipelines to move the data in real-time to derive actionable insights that inform important business decisions. However, there are major challenges in building and managing telemetry data pipelines, even if they are indispensable.
Agentic AI solves for all these challenges and is capable of delivering greater efficiency in managing and optimizing telemetry data pipeline health. An agentic AI can –
- Discover, deploy, and integrate with new data sources instantly;
- Parse and normalize raw data from structured and unstructured sources;
- Track and monitor pipeline health; be modular and sustain loss-less data flow;
- Identify and quarantine sensitive and PII data instantly;
- Manage and fix for schema drift and data quality;
- Segregate and evaluate data for storage in long-term storage, data lakes, or SIEM/observability tools
- Automate the transformation of data into different formats for different destinations;
- Save engineering team bandwidth which can be deployed on more strategic priorities
Curious about how agentic AI can solve your data problems? Get in touch with us to explore Cruz, our agentic AI data-engineer-in-a-box to solve your telemetry data challenges.
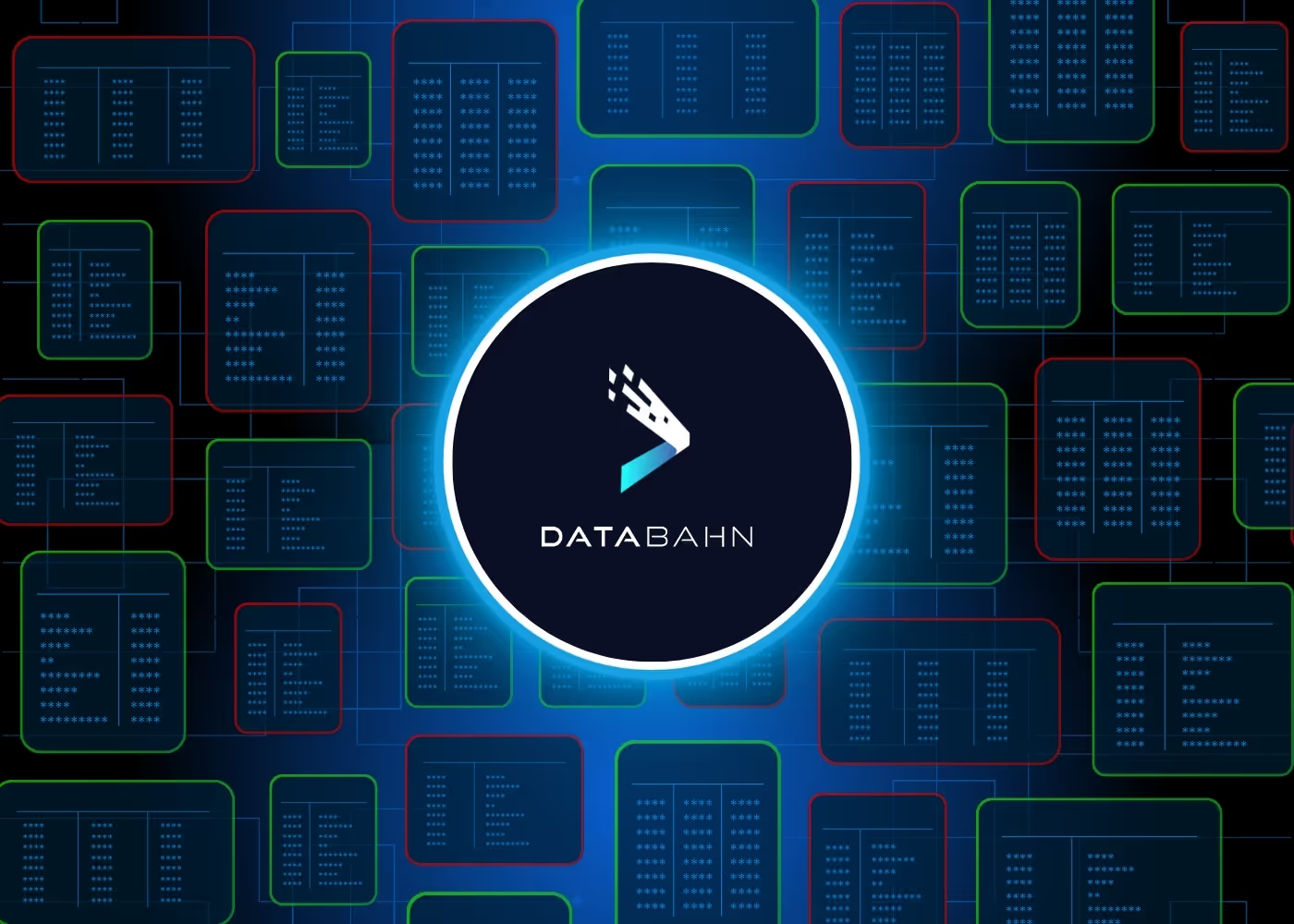
Reduce Alert Fatigue in Microsoft Sentinel
AI-powered log prioritization delivers 50% log volume reduction
Microsoft Sentinel has rapidly emerged as the preferred SIEM for enterprises seeking robust security monitoring and advanced threat detection. Its powerful analytics, integration with Microsoft products, and automation features make it an invaluable asset for security operations. However, as organizations connect more diverse data sources to gain complete visibility, they face a growing challenge of data overload. Security teams are increasingly overwhelmed by this surge in data, resulting in significant alert fatigue, escalating ingestion costs, and higher risks of critical threats going undetected. Reducing alert fatigue is a major priority for security leaders today.
The Alert Overload Reality in Sentinel
Sentinel excels at integrating Microsoft data sources, allowing security teams to connect Azure, Office 365, and other Microsoft products with minimal effort. The challenge emerges when incorporating non-Microsoft sources, such as firewalls, network sources, and custom applications, which requires creating custom integrations and managing complex data pipelines. This process typically requires 4 to 8 weeks of engineering effort, which puts a strain on SOCs already stretched thin.
Faced with these integration hurdles and soaring costs, enterprises often take the expedient approach to route all logs into Sentinel without proper filtering or classification. This creates gaps in security visibility and threat detection and response, putting organizations at risk of undetected security incidents. As data volumes grow exponentially, security teams paradoxically find themselves caught in a frustrating cycle: more data means more alerts, which requires more analysts, which demands more budget—all while actual security outcomes deteriorate.
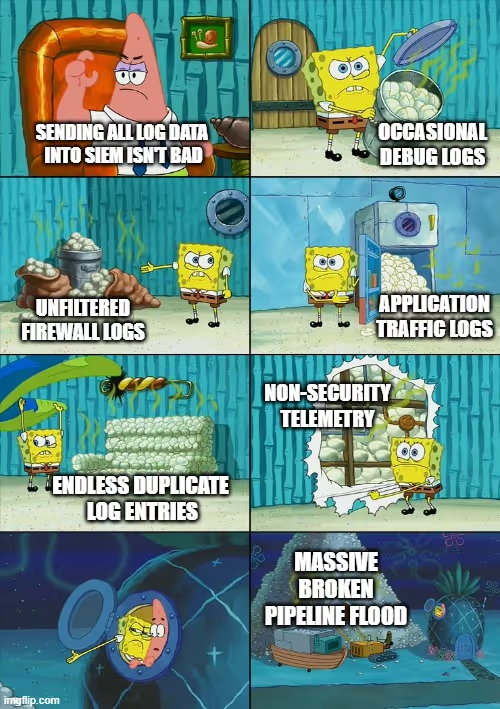
Why Traditional Log Management Hampers Sentinel Performance
The conventional approach to log management struggles to scale with modern security demands as it relies on static rules and manual tuning. When unfiltered data floods Sentinel, analysts find themselves filtering out noise and managing massive volumes of logs rather than focusing on high-priority threats. Diverse log formats from different sources further complicate correlation, creating fragmented security narratives instead of cohesive threat intelligence.
Without this intelligent filtering mechanism, security teams become overwhelmed, significantly increasing false positives and alert fatigues that obscures genuine threats. This directly impacts MTTR (Mean Time to Respond), leaving security teams constantly reacting to alerts rather than proactively hunting threats.
The key to overcoming these challenges lies in effectively optimizing how data is ingested, processed, and prioritized before it ever reaches Sentinel. This is precisely where DataBahn’s AI-powered data pipeline management platform excels, delivering seamless data collection, intelligent data transformation, and log prioritization to ensure Sentinel receives only the most relevant and actionable security insights.
AI-driven Smart Log Prioritization is the Solution

Reducing Data Volume and Alert Fatigue by 50% while Optimizing Costs
By implementing intelligent log prioritization, security teams achieve what previously seemed impossible—better security visibility with less data. DataBahn's precision filtering ensures only high-quality, security-relevant data reaches Sentinel, reducing overall volume by up to 50% without creating visibility gaps. This targeted approach immediately benefits security teams by significantly reducing alert fatigues and false positives as alert volume drops by 37% and analysts can focus on genuine threats rather than endless triage.
The results extend beyond operational efficiency to significant cost savings. With built-in transformation rules, intelligent routing, and dynamic lookups, organizations can implement this solution without complex engineering efforts or security architecture overhauls. A UK-based enterprise consolidated multiple SIEMs into Sentinel using DataBahn’s intelligent log prioritization, cutting annual ingestion costs by $230,000. The solution ensured Sentinel received only security-relevant data, drastically reducing irrelevant noise and enabling analysts to swiftly identify genuine threats, significantly improving response efficiency.
Future-Proofing Your Security Operations
As threat actors deploy increasingly sophisticated techniques and data volumes continue growing at 28% year-over-year, the gap between traditional log management and security needs will only widen. Organizations implementing AI-powered log prioritization gain immediate operational benefits while building adaptive defenses for tomorrow's challenges.
This advanced technology by DataBahn creates a positive feedback loop: as analysts interact with prioritized alerts, the system continuously refines its understanding of what constitutes a genuine security signal in your specific environment. This transforms security operations from reactive alert processing to proactive threat hunting, enabling your team to focus on strategic security initiatives rather than data management.
Conclusion
The question isn't whether your organization can afford this technology—it's whether you can afford to continue without it as data volumes expand exponentially. With DataBahn’s intelligent log filtering, organizations significantly benefit by reducing alert fatigue, maximizing the potential of Microsoft Sentinel to focus on high-priority threats while minimizing unnecessary noise. After all, in modern security operations, it’s not about having more data—it's about having the right data.
Watch this webinar featuring Davide Nigro, Co-Founder of DOTDNA, as he shares how they leveraged DataBahn to significantly reduce data overload optimizing Sentinel performance and cost for one of their UK-based clients.
.avif)
Microsoft Sentinel best practices
How SOCs can optimize Sentinel costs & performance
Enterprises and security teams are increasingly opting for Microsoft Sentinel for its comprehensive service stack, advanced threat intelligence, and automation capabilities, which facilitate faster investigations.
However, security teams are often caught off guard by the rapid escalation of data ingestion costs with Sentinel. As organizations scale their usage of Sentinel, the volume of data they ingest increases exponentially. This surge in data volume results in higher licensing costs, adding to the financial burden for enterprises. Beyond the cost implications, this data overload complicates threat identification and response, often resulting in delayed detections or missed signals entirely. Security teams find themselves constantly struggling to filter noise, manage alert volumes, and maintain operational efficiency while working to extract meaningful insights from overwhelming data streams.
The Data Overload Problem for Microsoft Sentinel
One of Sentinel's biggest strengths is its ease of integrating Microsoft data sources. SIEM operators can connect Azure, Office, and other Microsoft sources to Sentinel with ease. However, the challenge emerges when integrating non-Microsoft sources, which requires creating custom integrations and managing data pipelines.
For Sentinel to provide comprehensive security coverage and effective threat detection, all relevant security data must be routed through the platform. This requires connecting various security data sources such as firewalls, EDR/XDR, and even business applications to Sentinel, resulting in a 4 to 8 week data engineering effort that SOCs have to absorb.
On the other hand, enterprises often stop sending firewall logs to Sentinel due to the increasing log volume and costs associated with unexpected data volume spikes, which also lead to frequent breaks and issues in the data pipelines.
Then vs. Now: Key to Faster Threat Detection
Traditional data classification methods struggle to keep pace with modern security challenges. Security teams often rely on predefined rules or manual processes to categorize and prioritize data. As volumes expand exponentially, these teams find themselves ill-equipped to handle large data ingestions, resulting in critical losses of real-time insights!
DataBahn aids Sentinel deployments by streamlining data collection and ingestion with over 400 plug-and-play connectors. The platform intelligently defines data routing between basic and analytics tables while deploying strategic staging locations to efficiently publish data from third-party products into your Sentinel environment. With DataBahn’s volume reduction functions like aggregation and suppression to convert noisy logs like network traffic into manageable insights that can be loaded into Sentinel, effectively reducing both data volume and the overall time for query execution.
DOTDNA's AIDF Framework
DOTDNA has developed and promotes the Actionable Data Ingestion Framework (ADIF), designed to separate signal from noise by sorting your log data into two camps: critical, high-priority logs that are sent to Security Information and Event Management (SIEM) for real-time analysis and non-critical background data that can be stored long-term in cost-effectively storage.
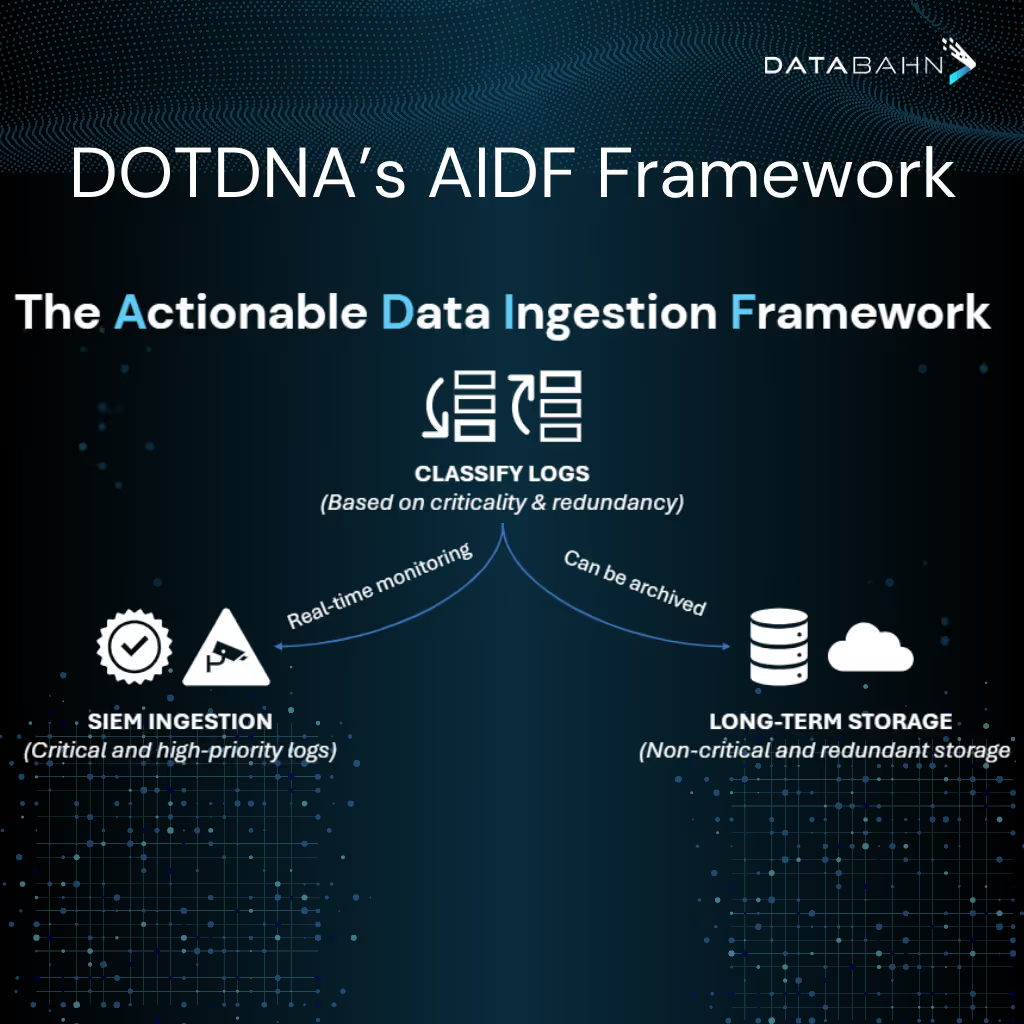
The framework streamlines log ingestion processes, prioritizes truly critical security events, eliminates redundancy, and precisely aligns with your specific security use cases. This targeted approach ensures your CyberOps team remains focused on high-priority, actionable data, enabling enhanced threat detection and more efficient response. The result is improved operational efficiency and significant cost savings. The framework guarantees that only actionable information is processed, facilitating faster investigations and better resource allocation.
The Real Impact
Following an acquisition, a UK-based enterprise needed to consolidate multiple SIEM and SOC providers into a single Sentinel instance while effectively managing data volumes and license costs. DOTDNA implemented DataBahn's Data Fabric to architect a solution that intelligently filters, optimizes, and dynamically tags and routes only security-relevant data to Sentinel, enabling the enterprise to substantially reduce its ingestion and data storage costs.
Optimizing Log Implementation via DOTDNA: Through the strategic implementation of this architecture, DOTDNA created a targeted solution that prioritizes genuine security signals before routing to Sentinel. This precision approach reduced the firm's ingestion and data storage costs by $230,000 annually while maintaining comprehensive security visibility across all systems.
Reduced Sentinel Ingestion Costs via DataBahn’s Data Fabric: The DataBahn Data Fabric Solution precisely orchestrates data flows, extracting meaningful security insights and delivering only relevant information to your Sentinel SIEM. This strategic filtering achieves a significant reduction in data volume without compromising security visibility, maximizing both your security posture and ROI.
Conclusion
As data volumes exponentially grow, DataBahn's Data Fabric empowers security teams to shift from reactive firefighting to proactive threat hunting. Without a modern data classification framework like ADIF, security teams risk feeling overwhelmed by irrelevant data, potentially leading to missed threats and delayed responses. Take control of your security data today with a strategic approach that prioritizes actionable intelligence. By implementing a solution that delivers only the most relevant data to your security tools, transform your security operations from data overload to precision threat detection—because effective security isn't about more data, it's about the right data.
This post is based on a conversation between Davide, Founder of DOTDNA with Databahn's CPO, Aditya Sundararam. You can view this conversation on LinkedIn here.
Subscribe to DataBahn blog!
Get expert updates on AI-powered data management, security, and automation—straight to your inbox

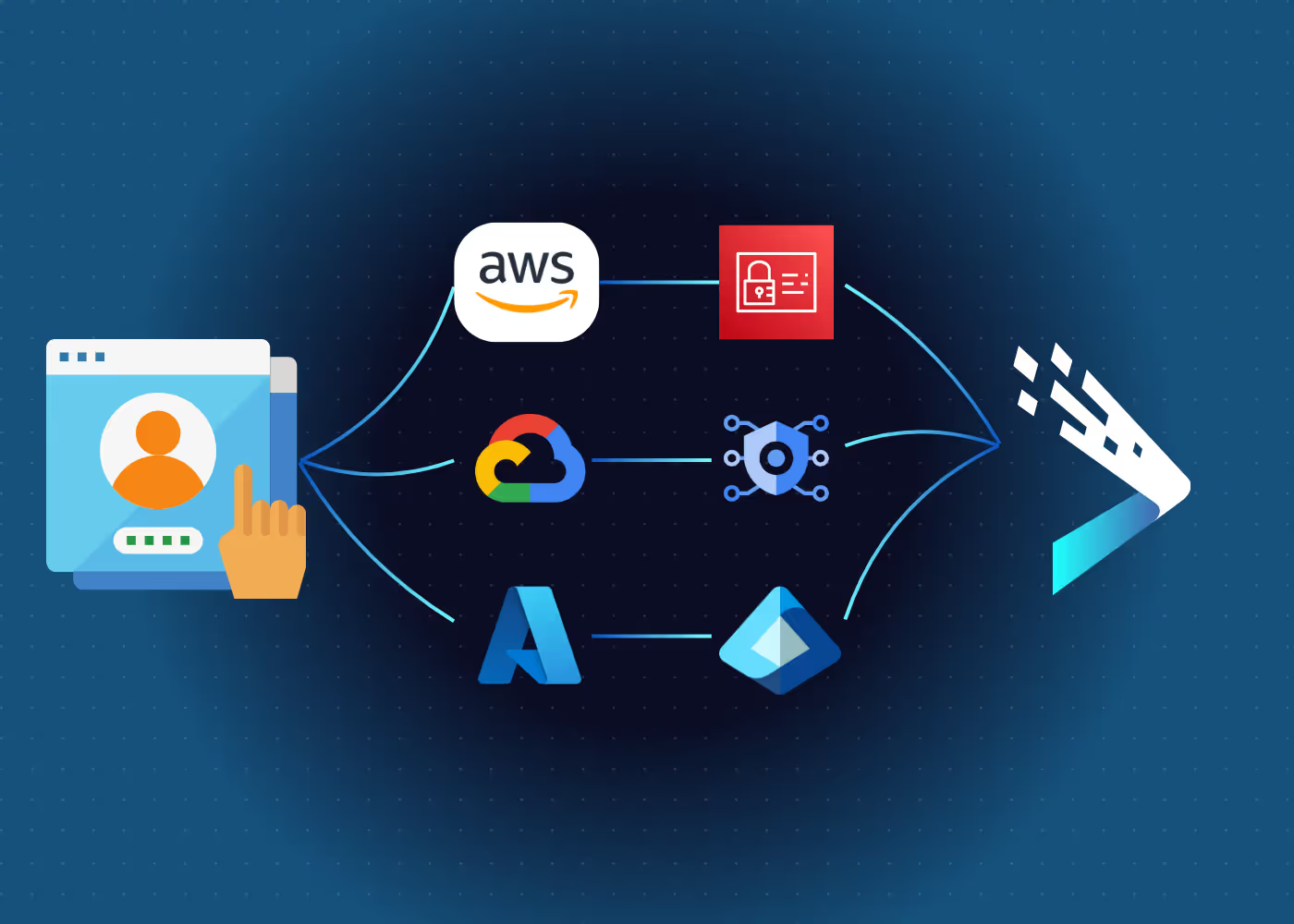
Identity Data Management
and how DataBahn solves the 'first-mile' identity data challenge
Identity management has always been about ensuring that the right people have access to the right data. With 93% of organizations experiencing two or more identity-related breaches in the past year – and with identity data fragmented and available in different silos – security teams face a broad ‘first-mile’ identity data challenge. How can they create a cohesive and comprehensive identity management strategy without unified visibility?
The Story of Identity Management and the ‘First-Mile’ data challenge
In the past, security teams would have to ensure that only a company’s employees and contractors had access to company data and to keep external individuals, unrecognized devices, and malicious applications out of organizational resources. This usually meant securing data on their own servers and restricting, monitoring, and managing access to this data.
However, two variables evolved rapidly to complicate this equation. First, several external users had to be provided access to some of this data as third-party vendors, customers, and partners needed to access enterprise data for business to continue functioning effectively. With new users coming in, existing standards and systems such as data governance, security controls, and monitoring apparatus did not evolve effectively to ensure consistency in risk exposure and data security.
Second, the explosive growth of cloud and then multi-cloud environments in digital enterprise data infrastructure has created a complex network of different identity and identity data collecting systems: HR platforms, active directories, cloud applications, on-premise solutions, and third-party tools. This makes it difficult for teams and company leadership to get a holistic view of user identities, permissions, and entitlements – without which, enforcing security policies, ensuring compliance, and managing access effectively becomes impossible.
This is the ‘First-Mile’ data challenge. How can enterprise security teams stitch together identity data from a tapestry of different sources and systems, stored in completely different formats, and enabling them to be easily leveraged for governance, auditing, and automated workflows?
How DataBahn’s Data Fabric addresses the ‘First-Mile’ data challenge
The ‘First-Mile’ data challenge can be broken down into 3 major components -
- Collecting identity data from different sources and environments into one place;
- Aggregating and normalizing this data into a consistent and accessible format; and
- Storing this data for easy reference, smart governance-focused and compliance-friendly storage.
When the first-mile identity data challenge is not solved, organizations face gaps in visibility, increase risks live privilege creep, and are vulnerable to major inefficiencies in identity lifecycle management, including provisioning and deprovisioning access.
DataBahn’s data fabric addresses the “first-mile” identity data challenge by centralizing identity, access, and entitlement data from disparate systems. To collect identity data, the platform enables seamless and instant no-code integration to add new sources of data, making it easy to connect to and onboard different sources, including raw and unstructured data from custom applications.
DataBahn also automates the parsing and normalization of identity data from different sources, pulling all the different data in one place to tell the complete story. Storing this data with the data lineage, multi-source correlation and enrichment, and the automated transformation and normalization in a data lake makes it easily accessible for analysis and compliance. With this in place, enterprises can have a unified source of truth for all identity data across platforms, on-premise systems, and external vendors in the form of an Identity Data Lake.
Benefits of a DataBahn-enabled Identity Data Lake
A DataBahn-powered centralized identity framework empowers organizations with complete visibility into who has access to what systems, ensuring that proper security policies are applied consistently across multi-cloud environments. This approach not only simplifies identity management, but also enables real-time visibility into access changes, entitlements, and third-party risks. By solving the first-mile identity challenge, a data fabric can streamline identity provisioning, enhance compliance, and ultimately, reduce the risk of security breaches in a complex, cloud-native world.

Enabling smarter and more efficient analytics for Salesforce customers
As the world's leading customer relationship management (CRM) platform, Salesforce has revolutionized the way businesses manage customer relationships and has become indispensable for companies of all sizes. It powers 150,000 companies globally, including 80% of Fortune 500 corporations, and boasts a 21.8% market share in the CRM space - more than its four leading competitors combined. Salesforce becomes the central repository of essential and sensitive customer data, centralizing data from different sources. For many of their customers, Salesforce becomes the single source of truth for customer data, which includes critical transactional and business-critical data with significant security and compliance relevance.
Business leaders need to analyze transaction data for business analytics and dashboarding to enable data-driven decision-making across the organization. However, analyzing Salesforce data (or any other SaaS application) requires significant manual effort and places constraints on data and security engineering bandwidth.
We were able to act as an application data fabric and help a customer optimize Salesforce data analytics and auditing with DataBahn.
How DataBahn's application data fabric enables faster and more efficient real-time analytics Read More
Why is auditing important for Salesforce?
How are auditing capabilities used by application owners?
SaaS applications such as Salesforce have two big auditing use cases - transaction analysis for business analytics reporting and security monitoring on application access. Transaction analysis on Salesforce data is business critical and is often used to build dashboards and analytics for the C-suite to evaluate key outcomes such as sales effectiveness, demand generation, pipeline value, and potential, customer retention, customer lifetime value, etc. Aggregating data into Salesforce to track revenue generation and associated metrics, and translating them into real-time insights, drives essential data-driven decision-making and strategy for organizations. From a security perspective, it is essential to effectively manage and monitor this data and control access to it. Security teams have to monitor how these applications and the underlying data are accessed, and the prevalent AAA (Authentication, Authorization, and Accounting) framework necessitates a detailed security log and audit to protect data and proactively detect threats.
Why are native audit capabilities not enough?
While auditing capabilities are available, using them requires considerable manual effort. Data needs to be imported manually to be usable for dashboarding. Additionally, data retention windows in these applications natively are short and are not conducive for comprehensive analysis, which is required for both business analytics and security monitoring. This means that data needs to be manually exported from Salesforce or other applications (individual audit reports), cleaned up manually, and then exported to a data lake to perform analytics. Organizations can explore solutions like Databricks or Amazon Security Lake to improve visibility and data security across cloud environments.
Why is secured data retention for auditing critical?
Data stored in SaaS applications is increasingly becoming a target for malicious actors given its commercial importance. Ransomware attacks and data breaches have become more common, and a recent breach in Knowledge Bases for a major global SaaS application is a wake-up call for businesses to focus on securing the data they store in SaaS applications or export from it.
DataBahn as a solution
DataBahn acts as an application data fabric, a middleware solution. Using DataBahn, businesses can easily fork data to multiple consumers and destinations, reducing data engineering effort and ensuring that high-quality data was being sent to wherever it needed to be for both simple (storage) or higher-order functions (analytics and dashboards). With a single-click integration, DataBahn prepares SaaS application data from Salesforce or a variety of other SaaS applications - Servicenow, Okta, etc. available for business analytics and security threat detection.
Using DataBahn also helps businesses more efficiently leverage a data lake, a BI solution, or SIEM. The platform enriches data and enables transformation into different relevant formats without manual effort. Discover these and other benefits of using an Application Data Fabric to collect, manage, control, and govern data movement.

Introducing Cruz: An AI Data Engineer In-a-Box
Why we built it and what it does
Artificial Intelligence is perceived as a panacea for modern business challenges with its potential to unlock greater efficiency, enhance decision-making, and optimize resource allocation. However, today’s commercially-available AI solutions are reactive – they assist, enhance analysis, and bolster detection, but don’t act on their own. With the explosion of data from cloud applications, IoT devices, and distributed systems, data teams are burdened with manual monitoring, complex security controls, and fragmented systems that demand constant oversight. What they really need is more than an AI copilot, but a complementary data engineer that takes over all the exhausting work and freeing them up for more strategic data and security work.
That’s where we saw an opportunity. The question that inspired us: How do we transform the way organizations approach data management? The answer led us to Cruz—not just another AI tool, but an autonomous AI data engineer that monitors, detects, adapts, and actively resolves issues with minimal human intervention.
Why We Built Cruz
Organizations face unprecedented challenges in managing vast amounts of data across multiple systems. From integration headaches to security threats, data engineers and security teams are under immense pressure to keep pace with evolving data risks. These challenges extend beyond mere volume—they strike at the effectiveness, security, and real-time insight generation.
- Integration Complexity
Data ecosystems are expanding, encompassing diverse tools and platforms—from SIEMs to cloud infrastructure, data lakes, and observability tools. The challenge lies in integrating these disparate systems to achieve unified visibility without compromising security or efficiency. Data teams often spend days or even weeks developing custom connections, which then require continuous monitoring and maintenance.
- Disparate Data Formats
Data is generated in varied formats—from logs and alerts to metrics and performance data—making it difficult to maintain quality and extract actionable insights. Compounding this challenge, these formats are not static; schema drifts and unexpected variations further complicate data normalization.
- The Cost of Scaling and Storage
With data growing exponentially, organizations struggle with storage, retrieval, and analysis costs. Storing massive amounts of data inflates SIEM and cloud storage costs, while manually filtering out data without loss is nearly impossible. The challenge isn’t just about storage—it’s about efficiently managing data volume while preserving essential information.
- Delayed and Inconsistent Insights
Even after data is properly integrated and parsed, extracting meaningful insights is another challenge. Overwhelming volumes of alerts and events make it difficult for data teams to manually query and review dashboards. This overload delays insights, increasing the risk of missing real-time opportunities and security threats.
These challenges demand excessive manual effort—updating normalization, writing rules, querying data, monitoring, and threat hunting—leaving little time for innovation. While traditional AI tools improve efficiency by automating basic tasks or detecting predefined anomalies, they lack the ability to act, adapt, and prioritize autonomously.
What if AI could do more than assist? What if it could autonomously orchestrate data pipelines, proactively neutralize threats, intelligently parse data, and continuously optimize costs? This vision drove us to build Cruz to be an AI system that is context-aware, adaptive, and capable of autonomous decision-making in real time.
Cruz as Agentic AI: Informed, Perceptive, Proactive
Traditional data management solutions are struggling to keep up with the complexities of modern enterprises. We needed a transformative approach—one that led us to agentic AI. Agentic AI represents the next evolution in artificial intelligence, blending sophisticated reasoning with iterative planning to autonomously solve complex, multi-step problems. Cruz embodies this evolution through three core capabilities: being informed, perceptive, and proactive.
Informed Decision-Making
Cruz leverages Retrieval-Augmented Generation (RAG), to understand complex data relationships and maintain a holistic view of an organization’s data ecosystem. By analyzing historical patterns, real-time signals, and organizational policies, Cruz goes beyond raw data analysis to make intelligent, autonomous decisions enhancing efficiency and optimization.
Perceptive Analysis
Cruz’s perceptive intelligence extends beyond basic pattern detection. It recognizes hidden correlations across diverse data sources, differentiates between routine fluctuations and critical anomalies, and dynamically adjusts its responses based on situational context. This deep awareness ensures smarter, more precise decisions without requiring constant human intervention.
Proactive Intelligence
Rather than waiting for issues to emerge, Cruz actively monitors data environments, anticipating potential challenges before they impact operations. It identifies optimization opportunities, detects anomalies, and initiates corrective actions autonomously while continuously evolving to deliver smarter and more effective data management over time.
Redefining Data Management with Autonomous Intelligence
Modern data environments are complex and constantly evolving, requiring more than just automation. Cruz’s agentic capabilities redefine how organizations manage data by autonomously handling tasks traditionally consuming significant engineering time. For example, when schema drift occurs, traditional tools may only alert administrators, but Cruz autonomously analyzes the data pattern, identifies inconsistencies, and updates normalization in real-time.
Unlike traditional tools that rely on static monitoring, Cruz actively scans your data ecosystem, identifying threats and optimization opportunities before they escalate. Whether it's streamlining data flows, transforming data, or reducing data volume, Cruz executes these tasks autonomously while ensuring data integrity.
Cruz's Core Capabilities
- Plug and Play Integration: Cruz automatically discovers data sources across cloud and on-prem environments, providing a comprehensive data overview. With a single click, Cruz streamlines what would typically be hours of manual setup into a fast, effortless process, ensuring quick and seamless integration with your existing infrastructure.
- Automated Parsing: Where traditional tools stop at flagging issues, Cruz takes the next step. It proactively parses, normalizes, and resolves inconsistencies in real time. It autonomously updates schemas, masks sensitive data, and refines structures—eliminating days of manual engineering effort.
- Real-time AI-driven Insights: Cruz leverages advanced AI capabilities to provide insights that go far beyond human-scale analysis. By continuously monitoring data patterns, it provides real-time insights into performance, emerging trends, volume reduction opportunities, and data quality enhancements, enabling better decision-making and faster data optimization.
- Intelligent Volume Reduction: Cruz actively monitors data environments to identify opportunities for volume reduction by analyzing patterns and creating rules to filter out irrelevant data. For example, it identifies irrelevant fields in logs sent to SIEM systems, eliminating data that doesn't contribute to security insights. Additionally, it filters out duplicate or redundant data, minimizing storage and observability costs while maintaining data accuracy and integrity.
- Automating Analytics: Cruz operates 24/7, continuously monitoring and analyzing data streams in real-time to ensure no insights are missed. With deep contextual understanding, it detects patterns, anticipates potential threats, and uncovers optimization. By automating these processes, Cruz saves engineering hours, minimizes human errors, and ensures data remains protected, enriched, and readily available for actionable insights.
Conclusion
Cruz is more than an AI tool—it’s an AI Data Engineer that evolves with your data ecosystem, continuously learning and adapting to keep your organization ahead of data challenges. By automating complex tasks, resolving issues, and optimizing operations, Cruz frees data teams from the burden of constant monitoring and manual intervention. Instead of reacting to problems, organizations can focus on strategy, innovation, and scaling their data capabilities.
In an era where data complexity is growing, businesses need more than automation—they need an intelligent, autonomous system that optimizes, protects, and enhances their data. Cruz delivers just that, transforming how companies interact with their data and ensuring they stay competitive in an increasingly data-driven world.
With Cruz, data isn’t just managed—it’s continuously improved.
Ready to transform your data ecosystem with Cruz? Learn more about Cruz here.

Data Pipeline Management and Security Data Fabrics
In the recent past, DataBahn has been featured in 3 different narratives focused on security data -
- Cybersecurity experts like Cole Grolmus (Strategy of Security) discussing how DataBahn's "Security Data Fabric" solution is unbundling security data collection from SIEMs (Post 1, Post 2)
- VCs such as Eric Fett of NGP Capital talking about how DataBahn's AI-native approach to cybersecurity was revolutionizing enterprise SOCs attempts to combat alert fatigue and escalating SIEM costs (Blog)
- Most recently, Allie Mellen, a Principal Analyst at Forrester, shouted DataBahn out as a "Data Pipeline Management" product focusing on security use cases. (LinkedIn Post, Blog)
Being mentioned by these experts is a welcome validation. It is also a recognition that we are solving a relevant problem for businesses – and for these mentions to come from these different sources represents the perspectives from which we can consider our mission.
What are these experts saying?
Allie’s wonderful article (“If You’re Not Using Data Pipeline Management for Security and IT, You Need To”) expertly articulates how SIEM spending is increasing, and that SIEM vendors haven’t created effective tools for log size reduction or routing since it “… directly opposes their own interests: getting you to ingest more data into their platform and, therefore, spend more money with them.”
This aligns with what Cole alluded to, when he stated reasons why "Security Data Fabrics” shouldn’t be SIEMs", pointing to this same conflict of interest. He added that these misaligned incentives spilled over into interoperability, where proprietary data formats and preferred destinations would promote vendor lock-in, which he had previously mentioned Security Data Fabrics were designed to overcome.
Eric’s blog was focused on identifying AI-native cybersecurity disrupters, where he identified DataBahn as one of the leading companies whose architecture was designed to leverage and support AI features, enabling seamless integration into their own AI assets to “ … overcome alert fatigue, optimize ingestion costs, and allocate resources to the most critical security risks.”
What is our point of view?
The reflections of these experts resonate with our conception of the problem we are trying to solve—SOCs and Data Engineering teams overwhelmed by the laborious task of data management and the prohibitive cost of the time and effort involved in overcoming it.
- SIEM ingest costs are too high. ~70% of the data being sent to SIEMs is not security-relevant. Logs have extra fields you don’t always need, and indexed data becomes 3-5x the original size. SIEM pricing data depends upon the volume of data being ingested and stored with them – which strains budgets and reduces the value that SOCs get from their SIEMs.
We deliver a 35%+ reduction in SIEM costs by reducing log sizes in 2-4 weeks – and our AI-enabled platform enables ongoing optimization to continue to reduce log sizes.
- SIEM being the source of data ingestion is also a problem. SIEMs are not very good at data ingestion. While some SIEM vendors have associated cloud environments (Sentinel, SecOps) with native ingestion tools, adding new sources – especially custom apps or sources with unstructured data – requires extensive data engineering effort and 4-8 weeks of integration. Additionally, managing these data pipelines is challenging, as these pipelines are single points of failure. Back pressure and spikes in data volumes can cause data loss.
DataBahn ensures loss-less data ingestion via a mesh architecture that ensures a secondary channel to ingest data in case of any blockage. It also tracks and identifies sudden changes in volume, helping to identify issues faster.
- Data Formats and Schemas are a challenge. SIEMs, UEBAs, Observability Tools, and different data storage destinations come with their proprietary formats and schemas, which add another manual task of data transformation onto data engineering teams. Proprietary formats and compliance requirements also create vendor lock-in situations, which add to your data team’s cost and effort.
We automate data transformation, ensuring seamless and effortless data standardization, data enrichment, and data normalization before forking the data to the destination of your choice.
Our solution is designed for specific security use cases, including a library of 400+ connectors and integrations and 900+ volume reduction rules to reduce SIEM log volumes, as well as support for all the major formats and schemas – which puts it ahead of generic DPM tools, something which Allie describes in her piece.
Cole has been at the forefront of conversations around Security Data Fabrics, and has called out that DataBahn has built the most complete platform/product in the space, with capabilities across integration & connectivity, data handling, observability & governance, reliability & performance, and AI/ML support.
Conclusion
We are grateful to be mentioned in these vital conversations about security data management and its future, and we appreciate the time and effort being spent by these experts to drive these conversations. We hope that this increases the awareness of Data Pipeline Management, Security Data Fabrics, and AI-native data management tools - a venn diagram we are pleased to be at the intersection of - and look forward to continue our journey in solving the problems that these experts have identified.

The Case for a Security Data Lake
Today’s business challenges require organizations to consume and store a vast quantity of raw security data in the form of logs, events, and datasets, as well as a substantial volume of security analytics information to support threat hunting and forensics. For some industries, there are regulatory and compliance requirements which also necessitate the storage of security data for extensive time periods. With organizations supporting a diverse set of users across geographies, managing multiple cloud-environments and on-premises networks, and supporting multiple devices – all while ensuring a centralized and cohesive security solution. In such cases, ensuring that all security data is collected and maintained in one accessible location is important. Enterprises are increasingly turning to security data lakes to perform this task.
Read how DataBahn helped a company forced to use 3 different SIEMs for data retention for compliance by giving them control of their own data DOWNLOAD
What is a Security Data Lake?
A security data lake is a central hub for storing, processing, and securing vast quantities of structured, unstructured, and semi-structured security-related data and information. By creating a specialized data repository for security data and threat intelligence, security data lakes enable organizations and their security teams to find, understand, and respond to cybersecurity threats more effectively.
Having a security data lake overcomes two distinct problems – security and access. IT and Security teams at organizations would want to ensure that their security data isn’t easily accessible and / or editable to ensure it isn’t modified or corrupted but ensure that it is quickly and easily accessible for SOCs for a faster MTTD / MTTR. Keeping all security-relevant data in a specific security data lake makes it easier for SOCs to access relevant logs and security information at a high velocity, while keeping it secure from malicious or unpermitted access.
How does a Security Data Lake work?
In traditional cybersecurity, legacy SIEMs were built to collect all security-related data from various sources in one place, and then analyze them by structuring the data into predefined schemas to flag anomalous patterns and identify potential threats through real-time analysis and examining historical data for patterns and trends. However, with the explosion in security data volume, legacy SIEMs struggled to identify relevant threats, while enterprises struggled with the ballooning SIEM costs.
Security Data Lakes emerged to solve this problem, becoming a scalable repository for all security data that could be connected to multiple cybersecurity tools so that threats could be identified, and incidents could be managed and documented easily. SOCs architect a network whereby security data is collected and ingested into the SIEM, and then stored in the Security Data Lake for analysis or threat hunting purposes.
Use cases of a Security Data Lake
- Easy and fast analysis of data across large time periods – including multiple years! – without needing to go to multiple sources due to the centralization of security data.
- Simplified compliance with easier monitoring and reporting on security data to ensure all relevant security data is stored and monitored for compliance purposes.
- Smarter and faster security investigations and threat hunting with centralized data to analyze, leading to improved MTTD and MTTR for security teams.
- Streamline access and security data management by optimizing accessibility and permissions for your security teams across hybrid environments.
Benefits of a Security Data Lake
As a centralized security data repository, there are numerous benefits to modern cybersecurity teams in leveraging a security data lake to manage and analyze security data. Here are some of the key advantages:
- Enhanced Visibility and Contextual Insights: Security Data Lakes allow organizations to seamlessly blend security data with business information that provides contextual value. This enables more informed assessments and quicker responses by providing greater visibility and deeper insights into potential threats for security teams.
- Cost-Effective Long-Term Storage: Security Data Lakes enable organizations to store large volumes of historical security data. This enables more effective threat hunting, helping cybersecurity teams identify anomalous behavior better and track incidents of concern without incurring very high SIEM licensing costs.
- Accelerated Incident Investigation: By supporting a high number of concurrent users and substantial computing resources, security data lakes facilitate rapid incident investigations to ensure faster detection and faster response. This enables security teams to deliver improved MTTD and MTTR.
- Advanced Security Analytics: Security Data Lakes provide a platform for advanced analytics capabilities, enabling collaboration between security and data science teams. This collaboration can lead to the development of sophisticated behavior models and machine learning analytics, enhancing the organization’s ability to detect and respond to threats.
- Scalability and Flexibility: As data volumes and attack surfaces grow, security data lakes offer the scalability needed to manage and analyze vast amounts of data, especially as the volume of security data continues to rise and becomes troublesome for SOCs to manage. Using a Security Data Lake makes it easier for organizations to collect security data from multiple sources across environments with ease.
- AI-Readiness for SOCs: Owning your own security data is critical for SOCs to integrate and adopt best-of-breed AI models to automate investigations and enhance data governance. Preparing aggregated security data for use in AI models and building applications and workflows that leverage AI to make SOCs more effective requires centralized data storage.
Read how DataBahn helped a company forced to use 3 different SIEMs for data retention for compliance by giving them control of their own data DOWNLOAD

What on earth is security data fabric, and why do we suddenly need one?
Every time I am at a security conference, a new buzzword is all over most vendors’ signage, one year it was UEBA (User Entity and Behavioral Analytics), next EDR (Endpoint Detection and Response), then XDR (Extended Detection and Response), then it was (ASM) Attack Surface Management. Some of these are truly new and valuable capabilities, some of these are rebranding of an existing capability. Some vendors have something to do with the new capability (i.e., buzzword), and some are just hoping to ride the wave of the hype. This year, we will probably hear a lot on GenAI and cybersecurity, and on the security data fabric. Let me tackle the latter in this article, with another article to follow soon on GenAI and Cybersecurity.
Problem Statement:
Many organizations are dealing with an explosion of security logs directed to the SIEM and other security monitoring systems, Terabytes of data every day!
- How to better manage the growing cost of the security log data collection?
- Do you know if all of this data clogging your SIEM storage has high security value?
- Are you collecting the most relevant security data?
To illustrate, here is an example of windows security events and a view on what elements have high security value compared to the total volume typically collected:

- Do you have genuine visibility into potential security log data duplication and underlying inconsistencies? Is your system able to identify missing security logs and security log schema draft fast enough for your SOC to avoid missing something relevant?
- As SIEM and security analytics capabilities evolve, how do to best decouple security log integration from SIEM and other threat detection platforms to allow not only easier migration to lasted technology but provide cost-effective and seamless access of this security data for threat hunting and other user groups?
- Major Next Gen SIEMs operate on a consumption-based model expecting end users to break down queries by data source and/or narrowed time range; which increases the total # of queries executed and increases your cost significantly!! Major Next-Gen SIEMs operate on a consumption-based model expecting end users to break down queries by data source and/or narrowed time range; which increases the total # of queries executed and increases your cost significantly!!
As security practitioners, we either accepted these issues as the cost of running our SOC, handled some of these issues manually, or hoped that either the cloud and/or SIEM vendors would one day have a better approach to deal with these issues, to no avail. This is why you need a security data fabric.
What is a Security Data Fabric (SDF)?
A data fabric is a solution that connects, integrates, and governs data across different systems and applications. It uses artificial intelligence and metadata automation to create flexible and reusable data pipelines and services. For clarity, a data fabric is simply a set of capabilities that allows you a lot more control of your data end to end, on how this data is ingested and where to forward it and stores it, in service of your business end goals, compared to just collecting and hoarding a heap of data in an expensive data lake, and hoping one day some use will come of it. The security data fabric is meant to tightly couple these principles with deep security expertise and the use of artificial intelligence to allow mastery of your security data and optimize your security monitoring investments and enable enhanced threat detection.
They key outcome of a security data fabric is to allow security teams to focus on their core function (i.e., threat detection) instead of spending countless hours tinkering with data engineering tasks, which means automation, seamless integration and minimal overhead on ongoing operations.

Components of a Security Data Fabric (SDF):
Smart Collection:
This is meant to decouple the collection of the security data logs from the SIEM/UEBA vendor you are using. This allows the ability to send the relevant security data to the SIEM/UEBA, sending a copy to a security data lake to create additional AI-enabled threat detection use cases (i.e., AI workbench) or to perform threat hunting, and send compliance-related logs to cold storage.
Why important?
- Minimize vendor lock-in and allow your system to leverage this data in various environments and formats, without needing to pay multiple times to use your own security data outside of the SIEM - particularly for requirements such as threat hunting and the creation of advanced threat-detection use cases using AI.
- Eliminate data loss with traditional SIEM log forwarders, syslog relay servers.
- Eliminate custom code/scripts for data collection.
- Reduced data transfer between cloud environments, especially in the case of having a hybrid cloud environment.
Security Data Orchestration:
This is where the security expertise in the security data fabric becomes VERY important. The security data orchestration includes the following elements:
- Normalize, Parse, and Transform: Apply AI and security expertise for seamless normalization, parsing, and transforming of security data into the format you need for ingestion into your SIEM/UEBA tool, such as OCSF, CEF, CIM, or to a security data lake, or other data storage solutions.
- Data Forking: Again, applying AI and security expertise to identify which security logs have the right fields and attributes that have threat detection value and should be sent to the SIEM, and which other logs should be sent straight to cold storage for compliance purposes, as an example.
- Data Lineage and Data Observability: These are well-established capabilities in data management tools. We are applying it here to security data, so we no longer need to wonder if the threat detection rule is not firing because the log source is dead/MIA or because there are no hits. Existing collectors do not always give you visibility for individual log sources (at the level of the Individual device and log attribute/telemetry). This capability solves this challenge.
- Data Quality: Ability to monitor and alert on schema drift and track the consistency, completeness, reliability, and relevance of the security data collected, stored, and used
- Data Enrichment: This is where you start getting exciting value. The security data fabric uses its visibility to all your security data with insights using advanced AI such as:
- Correlate with threat intel showing new CVEs or IoCs impacting your assets, here is how it looks in the MITRE Att&ck kill chain and provides a historical view of the potential presence of these indicators in your environment.
- Recommendations on new threat detection use cases to apply based on your threat profile.
Why important?
- Automation: At face value, existing tools promise some of these capabilities, but they usually need a massive amount of manual effort and deep security expertise to implement. This allows the SOC team to focus on their core function (i.e., threat detection) instead of spending countless hours tinkering with data engineering tasks.
- Volume Reduction: This is the most obvious value of using a security data fabric. You can reduce 30-50% of the data volume being sent to your SIEM by using a security-intelligent data fabric, as it will only forward data that has security value to your SIEM and send the rest to cheaper data storage. Yes, you read this correctly, 30-50% volume reduction! Imagine the cost savings and how much new useful security data you can start sending to your SIEM for enhanced threat detection.
- Enhanced Threat Detection: An SDF will enable the threat-hunting team to run queries more effectively and cheaply by giving them the ability to access a separate data lake, you get full control of your security data, and ongoing enrichments in how to improve your threat detection capabilities. Isn’t this what a security solution is about at the end of the day?